Cover image source: Tim Easley.
It’s easy to get off-track and start building the wrong product or selling the wrong ideas if you’re not constantly talking to your users and refreshing your understanding of what they need.
As you get bigger, it gets harder and harder to conduct productive user interviews. Talking to other human beings is a time-consuming process. It’s messy and often low-signal.
An effective UX research workflow amplifies that signal so the team can get insights faster.
The problem with your user interview workflow
Learning from user interviews is always an intuitive process.
As you talk to people, you begin to notice clusters of correlations between people and their usage of the product. You notice that a lot of feature X’s usage is from people with the title “director of marketing.” You notice that companies of 100 people or fewer tend to have trouble justifying the price point of your Pro plan.
You take these insights and plot them out, eventually forming groups of users with distinct needs, buying processes, or price sensitivities:
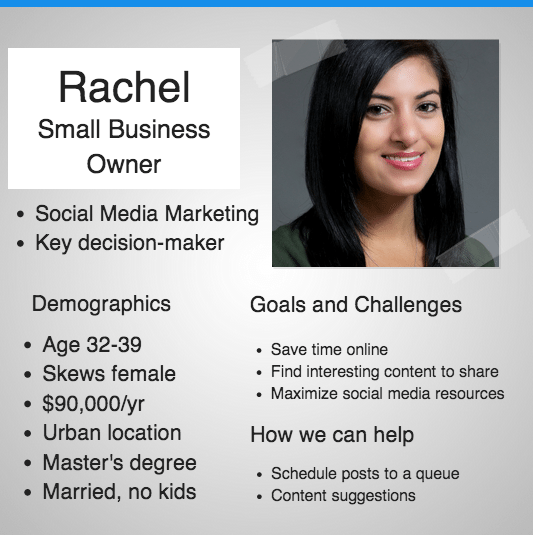
These personas are supposed to be used for everything from business development to sales to product management.
Too often, however, your user personas end up in a Google Doc that doesn’t get updated for months. It lays fallow while your team builds the product, solves problems, and readjusts. Startups often just don’t have the time to constantly redo the process of data collection and analysis that went into their personas the first time.
Many have even raised the question of whether user personas, as an idea, are simply dead. There are some big problems with them (or at least the way they’re commonly thought of today):
-
People are multidimensional, not schematic: You don’t get nuance when you’re defining people according to their title and salary.
-
Personas are static and fragile: Changes in your market and changes in your product will throw your user personas off track. Keeping them updated is a significant logistics and data challenge.
-
Too hard to explore data, too easy to introduce bias: You rely mainly on your instincts to figure out how people fit together. That introduces a huge opportunity for confirmation bias to seep into the process.
That said, the solution is not to ditch personas, but to flip around the process that produces them.
The user taxonomy
Getting productive information out of your user interviews starts with setting up a user taxonomy.
Before you fly a plane, you check all the instruments and make sure that everything is working properly. You need to know that while you’re in the air you’re going to be collecting all the information you need, and that all the feedback you receive from your dashboard will be accurate.
Here, you should be instrumenting your workflow so that you’re getting the maximum amount of useful data from each interaction with a user.
The same way Linnaean taxonomy lets us classify flora and fauna, a user taxonomy lets us classify the users we talk to according to demographic and behavioral attributes like:
-
age: how old they are
-
income: how much money they earn per year, estimated
-
products purchased: what products/services they’ve purchased in the past
This involves equal parts art and science — you need to know your product and your market in order to pick characteristics properly.
Here’s an example of the potential metadata around a user:
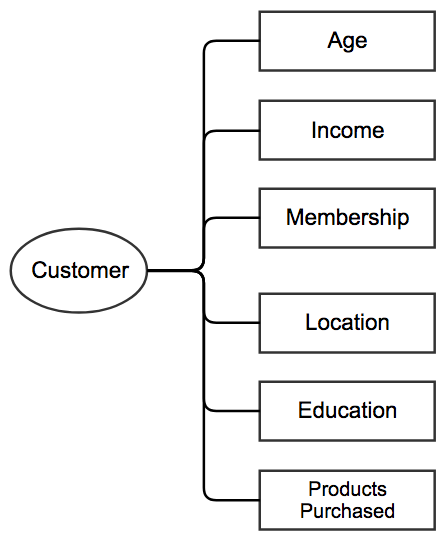
You probably collect this kind of information upon signup or through an integration with a social network like Google or Facebook.
As you do, you progressively build a profile of who your customers are:
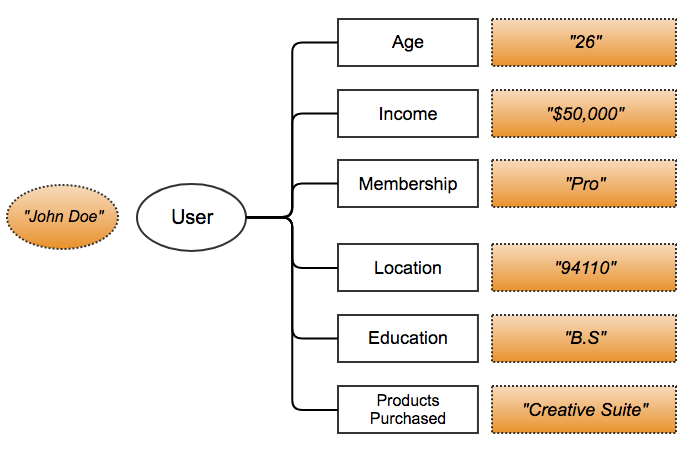
You can capture this type of information in a database like Airtable:
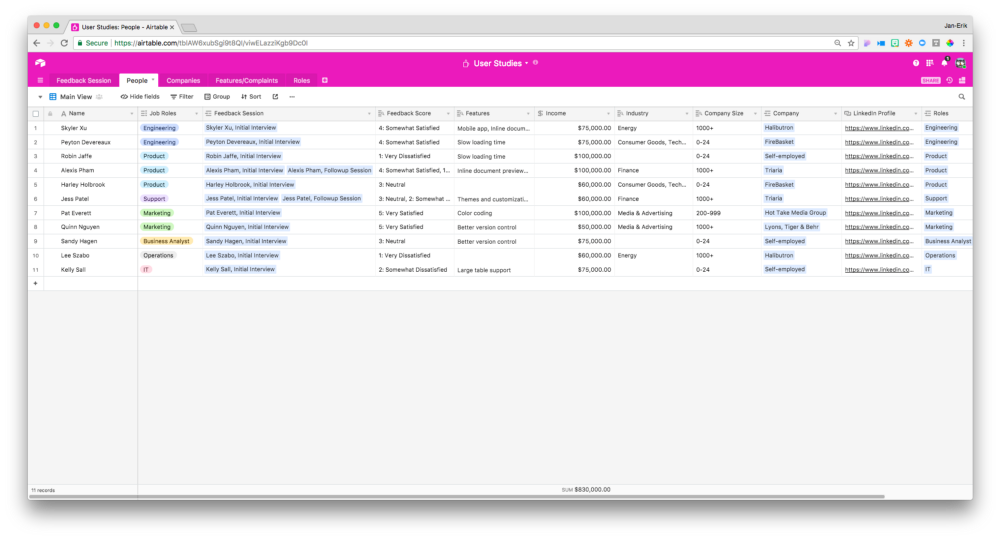
You can then take this one step further by adding a similar structure to your actual user interview process.
If you turn each question that you ask into an input, and turn each user’s answer to each into an output, then you can start supplementing your user profile with even more useful information.
This user taxonomy forms the foundation of your user interview pipeline. Next up is the process of collecting and filtering that information.
Collecting and filtering your information
Making your user interviews more productive is all about building a robust pipeline of data and then maximizing the number of ways you can look at it.
From the interview, capture additional metadata, like the date that the interview was performed, who the interviewer was, how much time was spent on each task, and so on:
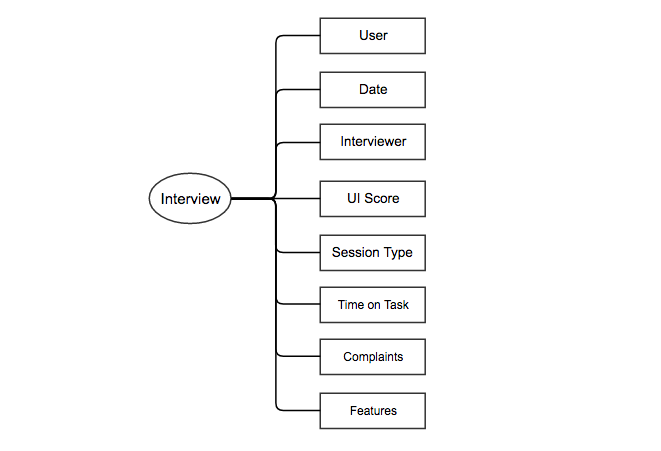
You can collect this interview metadata in a separate Feedback Session table in your Airtable base:
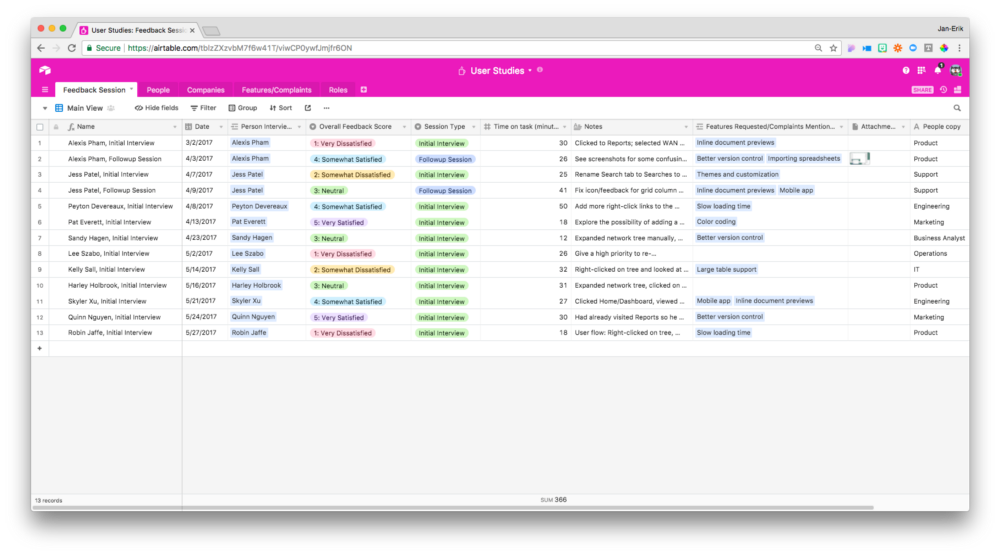
Although it’s in a separate table from your user metadata, you can connect each feedback session to the user who participated in it, linking your user and interview metadata and producing a definitive record of a user’s experience with your product.
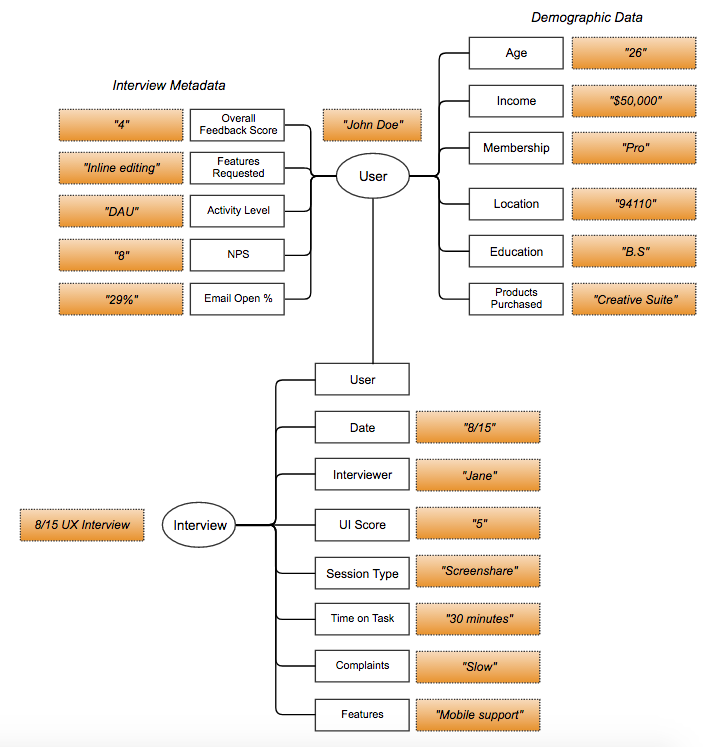
This linkage lets you view users in the context of everything from their demographic info to the kinds of answers they gave on user interviews, and enables you to create more fleshed-out user personas.
Filter your data by role, and you can see all your users’ characteristics in the context of the team they work on:
-
Average feedback score
-
Time taken on task
-
NPS
-
Company size
-
ARPU
If you find out that one type of team produces consistently higher ARPU users at much lower cost to you, that’s valuable information to consider when creating your messaging and working on your customer acquisition funnel.
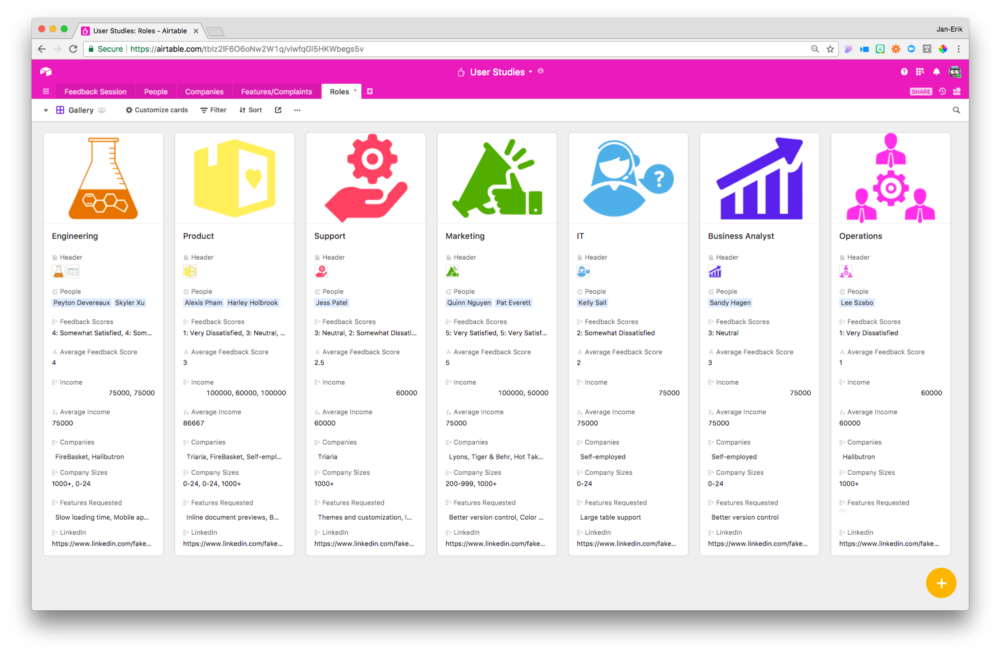
Building user personas, however, is just one application of a technique that we can use to develop many different types of insights.
Linking our user metadata with our interview metadata gives us the ability to understand our users from a large variety of different perspectives.
A user persona is just one perspective — you can have as many perspectives as types of metadata that you collect.
Filter your metadata on the fly
The reason you want to instrument your interview and user metadata like this is that you don’t always know the best way to analyze it right out of the gate.
It may seem more efficient to build a tool that automatically generates reports or spits out dashboards, but when you do that, you lose the power to flexibly filter through your data on the fly and explore different views of it.
Think of how you use Google when you’re doing research on a topic and don’t know precisely what you’re looking for. You craft your initial search query. You adjust; you change some words, add others. You change your search operators. You do this, over and over, until you find something interesting.
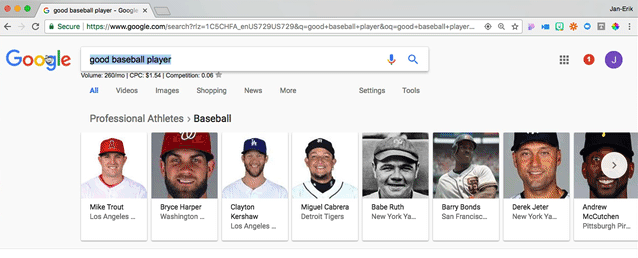
The process of discovering something worthwhile “starts with a vague idea about some gem that might be lurking in the data,” as Interana technologist Miroslav Klivansky says. Structuring the metadata from your user interviews allows you to follow up on all of your vague ideas.
You can take a specific interview session and view all of the metadata corresponding with the highest customer feedback scores that you received in order to understand, at a glance, the commonalities between all of your happy users. With just a few more clicks, you can instead view the metadata corresponding with the lowest customer feedback scores to see the commonalities between all of your unhappy users. This flexibility makes it easier to tease out useful insights from your data.
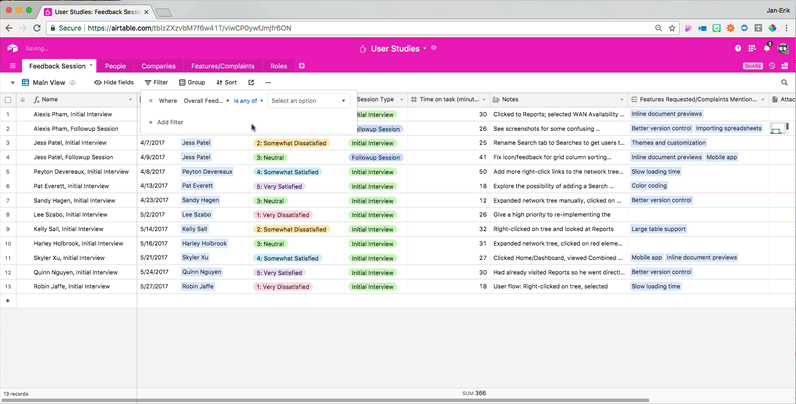
One way to use these views would be to look at how feature requests map to feedback scores. Users who rank themselves “very dissatisfied” can have urgent feature requests worth following up on quickly — unless their dissatisfaction is caused by the fundamental problem of a lack of product-user fit.
You can also filter by the interviews where your team spent the longest amount of time helping customers figure out how to do something, giving you insight into more problematic use cases for your product or customer groups that might need more attention:
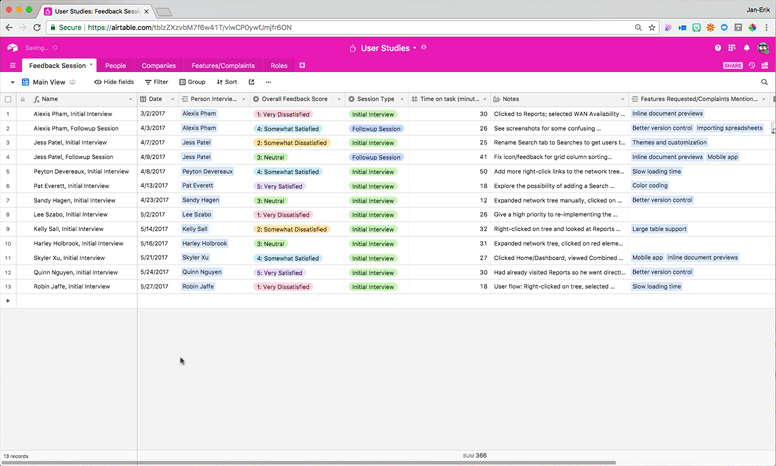
Another approach is to group your records by different feature requests. This lets you quickly gauge how many people want each feature on your roadmap, but it also lets you see which combinations of features people are most interested in and what kinds of users are asking for which features.
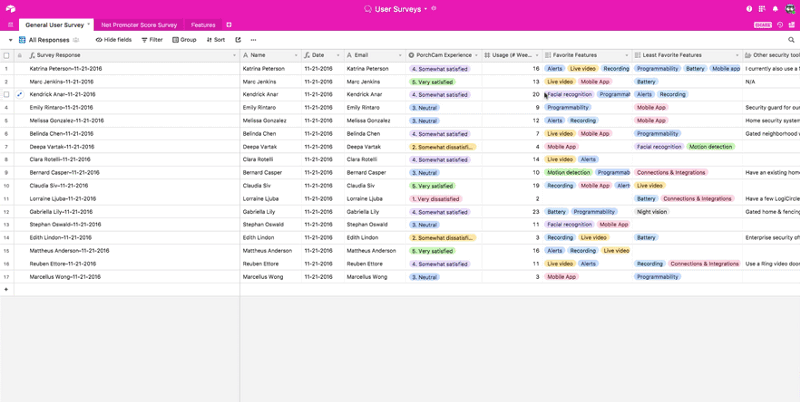
When you have this kind of extensive metadata structure, you can apply filters or group records based on your user interview and demographic metadata. Most importantly, in a tool like Airtable, you can do it quickly. All of those pieces of data are visible and directly manipulable—so filtering them becomes a trivial task.
Rapid questioning allows for rapid growth
Static user personas and NPS surveys can only get you so far when it comes to building a customer-centric product.
People are multidimensional, and understanding what they want you to build is only possible if you analyze their behavior from multiple dimensions — that is, if you both collect the right data and get the right structure in place for viewing it.
Check out some of our Airtable UX and user research templates here.